Causal reasoning is the process of identifying causality: the relationship between a cause and its effect. The study of causality extends from ancient philosophy to contemporary neuropsychology; assumptions about the nature of causality may be shown to be functions of a previous event preceding a later one. The first known protoscientific study of cause and effect occurred in Aristotle's Physics.[1] Causal inference is an example of causal reasoning.
Understanding cause and effect
Causal relationships may be understood as a transfer of force.[2] If A causes B, then A must transmit a force (or causal power) to B which results in the effect. Causal relationships suggest change over time; cause and effect are temporally related, and the cause precedes the outcome.[3]
Causality may also be inferred in the absence of a force, a less-typical definition.[4] A cause can be removal (or stopping), like removing a support from a structure and causing a collapse or a lack of precipitation causing wilted plants.
Humans can reason about many topics (for example, in social and counterfactual situations and in the experimental sciences) with the aid of causal understanding.[3] Understanding depends on the ability to comprehend cause and effect. People must be able to reason about the causes of others’ behavior (to understand their intentions and act appropriately) and understand the likely effects of their own actions. Counterfactual arguments are presented in many situations; humans are predisposed to think about “what might have been”, even when that argument has no bearing on the current situation.
Cause-and-effect relationships define categories of objects.[5] Wings are a feature of the category "birds"; this feature is causally interconnected with another feature of the category, the ability to fly.[5]
Traditionally, research in cognitive psychology has focused on causal relations when the cause and the effect are both binary values; both the cause and the effect are present or absent.[6][7] It is also possible that both the cause and the effect take continuous values. For example, turning the volume knob of a radio (as the cause) increases or decreases the sound intensity (as the effect). In these cases, the relation between the variables of the cause and the effect resembles a mathematical function in which change in the variable of the cause changes values in the variable of the effect. Human learning of such relations has been studied in the field of "Function Learning".[8][9][10]
Even so, it is well understood that physical applications of continuous mathematical models are not literally continuous in practice.[11] A knob on a radio does not take on an uncountably infinite number of possible values—it takes a finite number of possible values fully limited by the mechanical, physical, nature of the knob itself. There exists no one-to-one mapping between the continuous mathematics used for engineering applications and the physical product(s) produced by the engineering. Indeed, this is a prominent problem within Philosophy of Mathematics.[12][13] One possible answer to this open question is that reality is rasterized (possibly at the Planck Scale, see Loop Quantum Gravity) and is fundamentally discrete. So goes the theory of mathematical fictionalism, where continuous mathematics serves as a fictional construct of imagery used for reasoning geometrically via drawings and intuitive ideas of shapes absent of measurement data.
Cause and effect may also be understood probabilistically, via inferential statistics, where the distinction between correlation and causation is important. Just because two variables are correlated does not mean that one caused the other. For example, ice cream sales are correlated with the number of deaths due to drowning. This is not because ice cream causes drowning or because drowning deaths cause people to buy ice cream. Rather, it is because a third factor causes both. In this case, hot weather causes people both to buy ice cream and to go swimming, and the latter increasing the chances of drowning. These other possible causes that can account for the correlation between two variables are called confounding variables.
In this way, ascertaining cause and effect relations is quite hard and arguably impossible through statistical observation alone. Statistical studies can alleviate the problem by controlling for variables suspected to be confounders, but it is still possible that an observed correlation is caused by some uncontrolled-for factor. The scientific method is a solution to this problem. In a scientific experiment, the experimenters vary an independent variable and observe the changes in the dependent variable. As long as the independent variable is varied in a random way across the sample (e.g., in a medical study, half of the participants may be chosen randomly to receive the treatment, and the other half a placebo), there will be no confounding variables that cause both the change in the independent and dependent variables, since the independent variable is controlled by the experimenters.
Causality is an important question in modern physics. According to deterministic theories, any future event could in principle be predicted with perfect knowledge of the present, since one could precisely calculate what outcome would be caused by the present state of affairs. However, quantum mechanics has brought back the possibility of indeterministic events - events that are not determined by prior causes. Whether the outcomes of quantum-mechanical events are really indeterminstic is one of the biggest open problems in physics today and is part of the interpretation of quantum physics and its reconciliation with the causal structure of special relativity.
Theories of causality also play important roles in debates about free will. For example, if determinism is true, it implies that our actions are caused by prior events, which incompatibilists argue is inconsistent with free will. As a result, incompatibilists fall into two main camps: libertarians (not to be confused with political libertarians), who argue that human actions are not determined by prior causes, and hard determinists, who argue that free will does not exist. The main challenge for libertarian philosophers is to explain how human actions are caused, if they are not caused by prior events. Some cite quantum mechanics as evidence that human actions may not be deterministic.[14][15] In opposition to both libertarians and hard determinists are compatiblists, who argue that the existence of free will is compatible with determinism.
Inferring cause and effect
Humans are predisposed to understand cause and effect, making inferences bi-directionally. Temporal cues demonstrate causality.[16] When observing an event, people assume that things preceding the event cause it, and things following the event are effects of it.[17]
Coincidence of movement and spatial relationships are another way to infer cause and effect. If objects move together (or one object seems to initiate the movement of another), causality is inferred from that relationship.[18] Animacy may also be inferred from such relationships.
Causal reasoning may be activated almost automatically.[3][19] However, inferences about cause and effect do not always demonstrate understanding of mechanisms underlying causality; causality has been described as "cognitive illusion".[20] Much understanding of cause and effect is based on associations, without an understanding of how events are related to one another.[21]
A 2013 neuropsychology study[22] demonstrates that humans conform new information to old information. This suggests an inverted causal experience: cause must be attributed to effect a posteriori to understand the causal connection between agent and act. Friedrich Nietzsche argued against Aristotelian causality (that cause precedes effect) in The Will To Power.[23]
Humans understand cause and effect. Research suggests that other animals, such as rats[24] and monkeys,[25] may or may not understand cause and effect. Animals may use information about cause and effect to improve decision-making and make inferences about past and future events.[26] A constant which guides human reasoning and learning about events is causality.[27] Causal considerations are integral to how people reason about their environment.[3] Humans use causal cues and their related effects to make decisions and predictions and to understand mechanisms leading to change.[28]
Types of causal relationships
Several types of causal models are developed as a result of observing causal relationships: common-cause relationships, common-effect relationships, causal chains and causal homeostasis.[21]
- In common-cause relationships, a single cause has several effects:

- A virus is an example of a single cause resulting in several effects (fever, headache and nausea).
- In common-effect relationships, several causes converge in one effect:

- An increase in government spending is an example of one effect with several causes (reduced unemployment, decreased currency value, and increased deficit).
- In causal chains one cause triggers an effect, which triggers another effect:

- An example is poor sleep leading to fatigue, which leads to poor coordination.
- In causal homeostasis, causal relationships form a stable cycle or reinforcing mechanism:
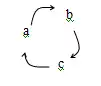
- Feathers, hollow bones, high metabolic rate and flight reinforce each other in birds, with adaptation to the whole rather than one instance beginning a causal relationship.[21]
Types of causal reasoning
While causal understanding can be automatic, in complex situations advanced reasoning is necessary. Types of causal reasoning[2] include:
Deduction
Deductive reasoning implies a general rule; an event is a guaranteed conclusion. An outcome may be deduced based on other arguments, which may determine a cause-and-effect relationship.
Induction
Inductive reasoning is an inference made with uncertainty; the conclusion is likely, but not guaranteed. Induction can be used to speculate about causality.
Abduction
In abductive reasoning, the premises do not guarantee a conclusion. Abduction moves from data description to a hypothesis without a necessary relationship between cause and effect.
Models
There are several models of how humans reason about causality.
Dependency
The dependency model asserts that effects are contingent upon causes;[3] cause and effect have a probable relationship.
Covariation
The covariation (regularity) model, a type of dependency model, suggests that humans understand relationships between causes and effects by their coincidence, inferring that change in a cause changes an effect.[29]
Mechanism
This model[2] suggests that cause and effect are mechanistically related. In this situation, there is a basic process underlying the cause and effect.
Dynamics
This model of causal representation[30] suggests that causes are represented by a pattern of forces. The force theory[31] is an extension of the dynamics model that applies to causal representation and reasoning (i.e., drawing inferences from the composition of multiple causal relations).
Development in humans
Children develop an ability to understand causality and make inferences based on cause and effect at an early age;[19] some research suggests that children as young as eight months can understand cause and effect.[32] An understanding of mechanism and causality go hand in hand; children need to understand cause and effect to understand the operation of mechanisms, which allows them to understand causal relationships.[21] Children ask "why?" at an early age to understand mechanism and, in turn, causality. A child's first "why" question often coincides with their first attempt to explain something, within the first year after acquiring language.[21] Children ask "why" to understanding mechanism and causality.[33]
The ability to understand and reason about causality at a young age allows children to develop naïve theories about many topics. Causality helps children learn about physics, language, concepts and the behavior of others.[19] There is a developmental pattern to the causal understanding children have.[33]
Infants have an understanding of causal power.[21] They know that certain causes have particular effects. Young children, from late infancy to early childhood, understand functional relations:[21] a particular property (or component of a mechanism) has a certain function. They also understand causal density: how causes can interact in a complex way.
Older children and adults continue to develop an understanding of mechanistic fragments.[21] They understand the components of a working system in isolation, although the full mechanistic details of a system do not emerge until adulthood. Jean Piaget defined preoperational, concrete operational and formal operational stages of development.
Across cultures
Causal attributions have been shown to be dissimilar among different cultures in several ways:
Causal attributions
Yan and Gaier[34] investigated causal attributions of college success and failure between two groups of students, American and Asian. The Asian group was from China, Korea, Japan and Southeast Asia. Performance was similar across the four nationalities. The students were asked to make judgments about someone else's successes and failures in schoolwork, and whether those outcomes were attributable to innate ability or to expended effort. American participants were much more likely to attribute academic achievement to ability than Asian participants were. Although Americans tended to rate success as attributable to effort, failure was not perceived as being a result of a lack of effort. Asian students did not show this pattern.
Comparisons between Western and Eastern children and adults suggest differences between the cultures in the causality attributable to particular illnesses.[35] After reading stories of illnesses and making inferences about the causes of those illnesses, both groups showed an understanding of the biological causes of most illnesses. However, all the children and the Eastern adults also attributed some illnesses (and their remedies) to magical causes.
Causal motivations
Members of individualist or collectivist cultures may make different attributions of the origins and motivations of movement on a small scale among animated objects, or what would cause movement within a group of animated objects.[36] Participants from the UK, China and Hong Kong were shown videos of animated fish on a computer screen. The videos depicted a central fish moving toward or away from a group of fish, and participants were asked to determine the relationship among the fish: internally motivated (the central fish was looking for food) or externally motivated (the central fish wanted to join the others). Another set of videos suggested that the group of fish was the predominant agent, with the individual fish being acted upon. These different videos provided an opportunity to determine whether group or individual action is the preferred motivating force among different cultures.
Self-reported results suggested that Asian participants preferred descriptions and situations where the group was the central focus and causal agent, while Westerners preferred situations in which the individual was the agent. These effects also extended to memory processes; collectivist participants had better memories of situations in which the group was primary. This suggests that members of individualistic cultures are more responsive to independent agents and members of collectivist cultures are more responsive when groups guide individual action.
Causal reasoning in non-human animals
Causal reasoning is not unique to humans; animals are often able to use causal information as cues for survival.[20] Rats are able to generalize causal cues to gain food rewards. Animals such as rats can learn the mechanisms required for a reward by reasoning about what could elicit a reward.[20]
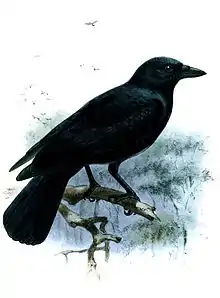
New Caledonian crows have been studied for their ability to reason about causal events. This intelligent species uses tools in a way that even chimpanzees cannot, making complex tools to bring food within reach.[37]
Experimental work with this species suggests that they can understand hidden causes in a way that was previously believed uniquely human.[38] In the first of two experiments a crow was confined, with food in a tube inaccessible to the crow without some effort. A human entered the enclosure and went behind a curtain, waving a stick near the food tube through a hole in the curtain. When the human left the enclosure the crow confidently moved toward the food area and retrieved the reward, knowing that the human cause of the moving stick (albeit invisible) was gone. In the second experiment, no human entered or exited the enclosure. In this case the crow moved toward the food uncertainly, not knowing what caused the stick to move.
See also
References
- ↑ Falcon, Andrea (2015-01-01). Zalta, Edward N. (ed.). The Stanford Encyclopedia of Philosophy (Spring 2015 ed.). Metaphysics Research Lab, Stanford University.
- 1 2 3 Ahn, W. K.; Kalish, C. W.; Medin, D. L.; Gelman, S. A. (1995-03-01). "The role of covariation versus mechanism information in causal attribution". Cognition. 54 (3): 299–352. doi:10.1016/0010-0277(94)00640-7. ISSN 0010-0277. PMID 7720361. S2CID 10841629.
- 1 2 3 4 5 Sloman, S. A. (2005). Causal models. New York, NY: Oxford University Press.
- ↑ Cheng, Patricia W.; Novick, Laura R. (1990). "A probabilistic contrast model of causal induction". Journal of Personality and Social Psychology. 58 (4): 545–567. doi:10.1037/0022-3514.58.4.545. PMID 2348358.
- 1 2 Rehder, B (2003). "Categorization as causal reasoning". Cognitive Science. 27 (5): 709–748. doi:10.1207/s15516709cog2705_2.
- ↑ Jenkins, H. M., & Ward, W. C. (1965). Judgment of contingency between responses and outcomes. Psychological Monographs, 79, ( 1, Whole No. 594).
- ↑ Cheng, P. W. (1997). From covariation to causation: A causal power theory. Psychological Review, 104, 367–405.
- ↑ Carroll, J. D. (1963). Functional learning: The learning of continuous functional mappings relating stimulus and response continua. NJ: Education Testing Service Princeton
- ↑ Brehmer, B. (1974). Hypotheses about relations between scaled variables in the learning of probabilistic inference tasks. Organizational Behavior and Human Performance, 11, 1–27.
- ↑ Koh, K., & Meyer, D. E. (1991). Function learning: Induction of continuous stimulus-response relations. Journal of Experimental Psychology: Learning, Memory, and Cognition, 17, 811–836.
- ↑ Maddy, Penelope (2017). Set-Theoretic Foundations
- ↑ Eklund, Matti (2019). Stanford Encyclopedia of Philosophy: Fictionalism
- ↑ Balaguer, Mark (2018). Stanford Encyclopedia of Philosophy: Fictionalism in the Philosophy of Mathematics
- ↑ Balaguer, Mark. (2010). MIT Press: Free Will as an Open Scientific Problem
- ↑ Hameroff, Stewart. (2012). Front Integr Neurosci.:How quantum brain biology can rescue conscious free will.
- ↑ Lagnado, David A.; Sloman, Steven A. (2006). "Time as a guide to cause". Journal of Experimental Psychology: Learning, Memory, and Cognition. 32 (3): 451–460. doi:10.1037/0278-7393.32.3.451. PMID 16719658. S2CID 16873453.
- ↑ Cheng, Patricia W.; Novick, Laura R. (1990). "A probabilistic contrast model of causal induction". Journal of Personality and Social Psychology. 58 (4): 545–567. doi:10.1037/0022-3514.58.4.545. PMID 2348358.
- ↑ Scholl, Brian J; Tremoulet, Patrice D (2000). "Perceptual causality and animacy". Trends in Cognitive Sciences. 4 (8): 299–309. doi:10.1016/s1364-6613(00)01506-0. PMID 10904254. S2CID 41797881.
- 1 2 3 Corrigan, Roberta; Denton, Peggy (1996-06-01). "Causal Understanding as a Developmental Primitive". Developmental Review. 16 (2): 162–202. doi:10.1006/drev.1996.0007.
- 1 2 3 Sawa, Kosuke (2009-09-01). "Predictive behavior and causal learning in animals and humans1". Japanese Psychological Research. 51 (3): 222–233. doi:10.1111/j.1468-5884.2009.00396.x. ISSN 1468-5884.
- 1 2 3 4 5 6 7 8 Keil, Frank C. (2006). "Explanation and Understanding". Annual Review of Psychology. 57 (1): 227–254. doi:10.1146/annurev.psych.57.102904.190100. ISSN 0066-4308. PMC 3034737. PMID 16318595.
- ↑ Kahan, Dan M.; Peters, Ellen; Dawson, Erica Cantrell; Slovic, Paul (2017). "Motivated numeracy and enlightened self-government". Behavioural Public Policy. Cambridge University Press. 1 (1): 54–86. doi:10.1017/bpp.2016.2. hdl:1794/18962. ISSN 2398-063X. S2CID 4694932. SSRN 2319992.
- ↑ Friedrich Nietzsche. The Will To Power. circa 1880s, which recent neuropsychological experiments confirm. 477 (Nov. 1887-March 1888) "I maintain the phenomenality of the inner world, too: every- thing of which we become conscious is arranged, simplified, schematized, interpreted through and through — the actual process of inner "perception," the causal connection between thoughts, feelings, desires, between subject and object, are absolutely hidden from us — and are perhaps purely imaginary. The "apparent inner world" is governed by just the same forms and procedures as the "outer" world. We never encounter "facts": pleasure and displeasure are subsequent and derivative intellectual phenomena — "Causality" eludes us; to suppose a direct causal link between thoughts, as logic does — that is the consequence of the crudest and clumsiest observation. Between two thoughts all kinds of affects play their game: but their motions are too fast, therefore we fail to recognize them, we deny them — "Thinking" as epistemologists conceive it, simply does not occur: it is a quite arbitrary fiction, arrived at by selecting one element from the process and eliminating all the rest, an artificial arrangement for the purpose of intelligibility — The "spirit," something that thinks: where possible even "absolute, pure spirit" – this conception is a second derivative of that false introspection which believes in "thinking": first an act is imagined which simply does not occur, "thinking," and secondly a subject-substratum in which every act of thinking, and nothing else, has its origin: that is to say, both the deed and the doer are fictions.
- ↑ Lovett, Richard A. (February 16, 2006). "Rats Understand Cause and Effect, Experiment Suggests". National Geographic News. National Geographic Society. Archived from the original on March 16, 2006. Retrieved 2016-12-27.
- ↑ Visalberghi, Elisabetta; Limongelli, Luca (1994). "Lack of comprehension of cause-effect relations in tool-using capuchin monkeys (Cebus apella)". Journal of Comparative Psychology. 108 (1): 15–22. doi:10.1037/0735-7036.108.1.15. PMID 8174341.
- ↑ Garcia-Retamero, Rocio; Wallin, Annika; Dieckmann, Anja (2007). "Does causal knowledge help us be faster and more frugal in our decisions?". Memory & Cognition. 35 (6): 1399–1409. doi:10.3758/BF03193610. ISSN 0090-502X. PMID 18035636.
- ↑ Sloman, Steven; A. Lagnado, David (2003-01-01). Motivation, BT - Psychology of Learning and (ed.). Causal Invariance in Reasoning and Learning. Psychology of Learning and Motivation. Vol. 44. Academic Press. pp. 287–325. doi:10.1016/s0079-7421(03)44009-7. ISBN 9780125433440.
- ↑ Hagmayer, Y., Sloman, S. A., Lagnado, D. A., & Waldmann, M. R. (2007). "Causal reasoning through intervention." In A. Gopnik & L. Schulz (Eds.), Causal learning: Psychology, philosophy, and computation (pp. 86–100). Oxford, England: Oxford University Press.
- ↑ Cheng, Patricia W. (1997). "From covariation to causation: A causal power theory". Psychological Review. 104 (2): 367–405. doi:10.1037/0033-295x.104.2.367. S2CID 6844972.
- ↑ Wolff, Phillip (2007). "Representing causation". Journal of Experimental Psychology: General. 136 (1): 82–111. doi:10.1037/0096-3445.136.1.82. PMID 17324086. S2CID 11303336.
- ↑ Wolff, Phillip; Barbey, Aron K. (2015-01-01). "Causal reasoning with forces". Frontiers in Human Neuroscience. 9: 1. doi:10.3389/fnhum.2015.00001. PMC 4301188. PMID 25653611.
- ↑ Sobel, David M.; Kirkham, Natasha Z. (2006). "Blickets and babies: The development of causal reasoning in toddlers and infants". Developmental Psychology. 42 (6): 1103–1115. doi:10.1037/0012-1649.42.6.1103. PMID 17087545. S2CID 11546585.
- 1 2 Keil, F. C. (2012-10-01). "Running on Empty? How Folk Science Gets By With Less". Current Directions in Psychological Science. 21 (5): 329–334. doi:10.1177/0963721412453721. S2CID 46741933.
- ↑ Yan, W.; Gaier, E. L. (2016-07-27). "Causal Attributions for College Success and Failure: An Asian-American Comparison". Journal of Cross-Cultural Psychology. 25 (1): 146–158. doi:10.1177/0022022194251009. S2CID 73591400.
- ↑ Nguyen, Simone P.; Rosengren, Karl S. (2004-03-01). "Causal Reasoning about Illness: A Comparison between European- and Vietnamese-American Children". Journal of Cognition and Culture. 4 (1): 51–78. doi:10.1163/156853704323074750. ISSN 1568-5373. S2CID 146247015.
- ↑ Ng, Sik Hung; Zhu, Ying (2001-04-01). "Attributing causality and remembering events in individual- and group-acting situations: A Beijing, Hong Kong, and Wellington comparison". Asian Journal of Social Psychology. 4 (1): 39–52. doi:10.1111/1467-839X.00074. ISSN 1467-839X.
- ↑ Hunt, Gavin R. (1996-01-18). "Manufacture and use of hook-tools by New Caledonian crows". Nature. 379 (6562): 249–251. Bibcode:1996Natur.379..249H. doi:10.1038/379249a0. S2CID 4352835.
- ↑ Taylor, Alex H.; Miller, Rachael; Gray, Russell D. (2012-10-02). "New Caledonian crows reason about hidden causal agents". Proceedings of the National Academy of Sciences. 109 (40): 16389–16391. Bibcode:2012PNAS..10916389T. doi:10.1073/pnas.1208724109. ISSN 0027-8424. PMC 3479607. PMID 22988112.